Artificial intelligence in healthcare is a relatively new and actively developing area of healthcare widely popular among doctors and scientists. The largest development is the employment of multimodal generative AI copilots for analyzing pathological conditions. The complex AI systems incorporate data inputs like medical images and data, clinical, and genetic data to improve the diagnosis of different diseases, particularly cancer. Generative AI development services integrated into Human Pathology Analysis have impacted the ability of pathologists to process complex data and arrive at efficient diagnoses.
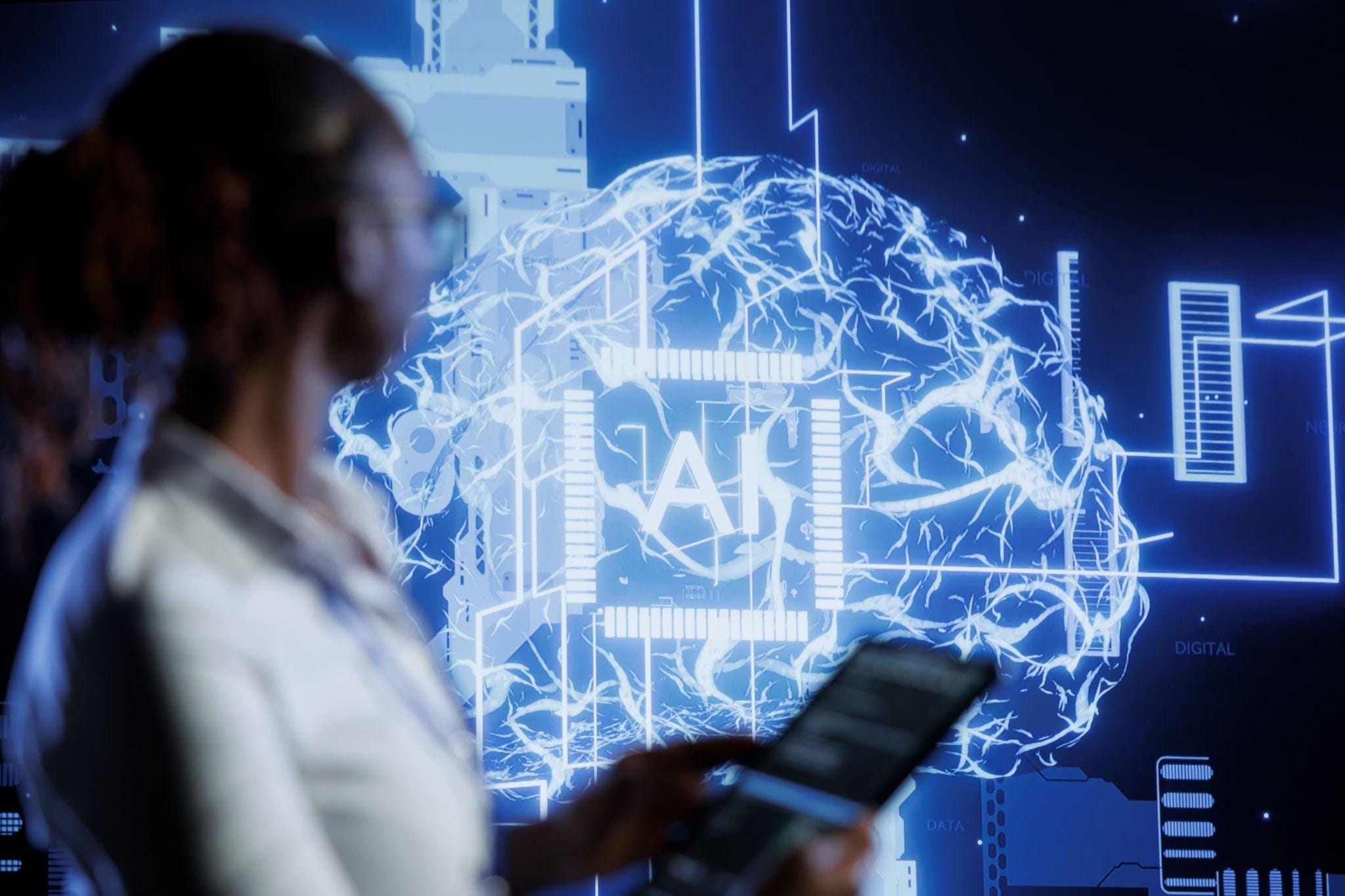
What is Multimodal Generative AI and how does it differ from using multiple modalities?
Multimodal generative AI can be defined as the ability of an AI system to work through and create data in various modalities. In the case of human pathology case analysis, these are developed to compile and analyze data from sources like digital pathology, radiology scans, patients’ records, genomic information, etc. Thus, providing additional valuable information to enlarge the information base for the pathologist’s decision and to make prompt decisions in patients’ treatment.
These AI systems incorporate generative models which is a type of model that can create new information from old information. This capability is most important for medical simulations in which the ability to simulate the progression of a disease or the efficacy of treatment can make all the difference for the patient. Multimodal generative AI development services and copilots are capable of handling extensive unstructured and heterogeneous information and offer insights that may not be obvious from one class of data.
For instance, multimodal AI systems can combine image analysis with genomic data to identify biomarkers associated with specific types of cancer. This integration enables pathologists to receive more comprehensive reports, which can improve diagnostic accuracy and allow for personalized treatment plans tailored to the genetic makeup of the tumor or disease.
Improving Diagnostic Accuracy and Efficiency
One of the main challenges in pathology is the risk of human error due to the high volume of data that needs to be analyzed. Multimodal generative AI copilots address this issue by providing more accurate and objective analyses of pathology slides and other patient data. These AI systems can detect subtle patterns or anomalies that might be overlooked by human pathologists, ensuring that diagnoses are both accurate and timely.
The use of AI also reduces the time required for diagnosis. In traditional pathology settings, pathologists may need to spend hours reviewing slides and medical records, but with the assistance of AI copilots, this process can be expedited. Multimodal AI systems can analyze large datasets in a fraction of the time it would take a human, enabling faster decision-making and reducing the time patients wait for their results.
Promising generative AI to improve Precision Medicine
The other benefit of applying multimodal generative AI in pathology is the aspect of precision medicine. Precision medicine implies that the process of establishing a patient’s treatment should be made according to the patient’s biochemical markers, genetics, habits, and exposure to other stimuli. Multimodal AI copilots contribute to the working possibility of precision medicine as they can gather and process data from different sources: from gene and image analysis.
For instance, AI copilots can help identify specific mutational patterns or genetic biomarkers associated with those diseases by analyzing genomic information to complement pathology scans of a patient. From it, doctors can provide patients with treatment options that are specific to their genetic profile and could respond positively to hence making them effective. With the help of multimodal AI systems, pathologists and clinicians will have the capabilities to diagnose and recommend a better care plan resulting in a better health outcome for patients.
Gather and Organise data to enhance the efficiency of its process.
The combination of various sources of information is one of the greatest difficulties of present-day medicine. Different types of data can be analyzed, such as histopathology images, clinical notes, lab results and genetic information and most of them are in different formats and different databases. Multimodal generative AI copilots solve this problem directly because they are designed to assimilate data efficiently.
It pointed out that such AI systems could pre-identify useful information and compile it from different sources into a single place. The latter not only optimizes the pathologists’ work but also guarantees the consideration of all the obtained data. By cutting down on data organization and data input, multimodal AI copilots allow pathologists more time for overall decision-making, report analysis, etc.
Practical Uses of Multimodal Artificial Intelligence in Pathology
Multimodal generative AI co-pilots in human pathology are no longer things of the future but are already being experienced in practice. These AI systems have been enlisted by several healthcare institutions and research organizations to boost pathology analysis. For instance, there are intelligent technologies in healthcare to identify cancer of various types, such as breast cancer, lung cancer, or prostate cancer.
Moreover, the use of multimodal artificial intelligence in analyzing digitized pathology slides as well as medical imaging data to detect early indicators of diseases to promote early diagnosis is being applied. These tools are also being used in the management of chronic diseases for instance diabetes whereby through the consistent analysis of medical information, the progression of the disease is managed.
Multimodal Generative AI: Review and Perspective of the Future
Currently, there are numerous potentials for Multimodal Generative AI, especially in pathology, and more uses are anticipated to be added in the subsequent years. What happens is that as the years go by and the technology behind artificial intelligence advancements is more refined, the systems will be able to give more insightful data about underlying diseases. Hence, the implementation of multimodal AI in pathology as a key medical area for the advancement of personalized medicine based on the use of genomic data will be one of the priorities of future healthcare.
In addition, since AI models can teach and update from a large database, they are likely to develop a better understanding of the characteristics of a problem and its ability to predict the results in diagnosing. That is why it is possible to consider that shortly, the role of multimodal AI systems in pathology will only increase and the work on the creation of such systems will accelerate even more as artificial intelligence opens new opportunities for the diagnostics, treatment and further management of diseases.
Conclusion
Multimodal generative AI copilots in human pathology analysis are changing the field for real by increasing accurate results, defining better precision medicine, and improving the overall performance of daily tasks. These AI systems combine data of different types and include modern patient data, imaging data, and clinical histories, so these are valuable instruments for pathologists. With the advancement of new AI technologies, their utilization in pathology will be even more crucial in the development of diagnosis and the subsequent care for the patients.
Author Bio
Anand Subramanian is a technology expert and AI enthusiast currently leading the marketing function at Intellectyx, a Data, Digital, and AI solutions provider with over a decade of experience working with enterprises and government departments.