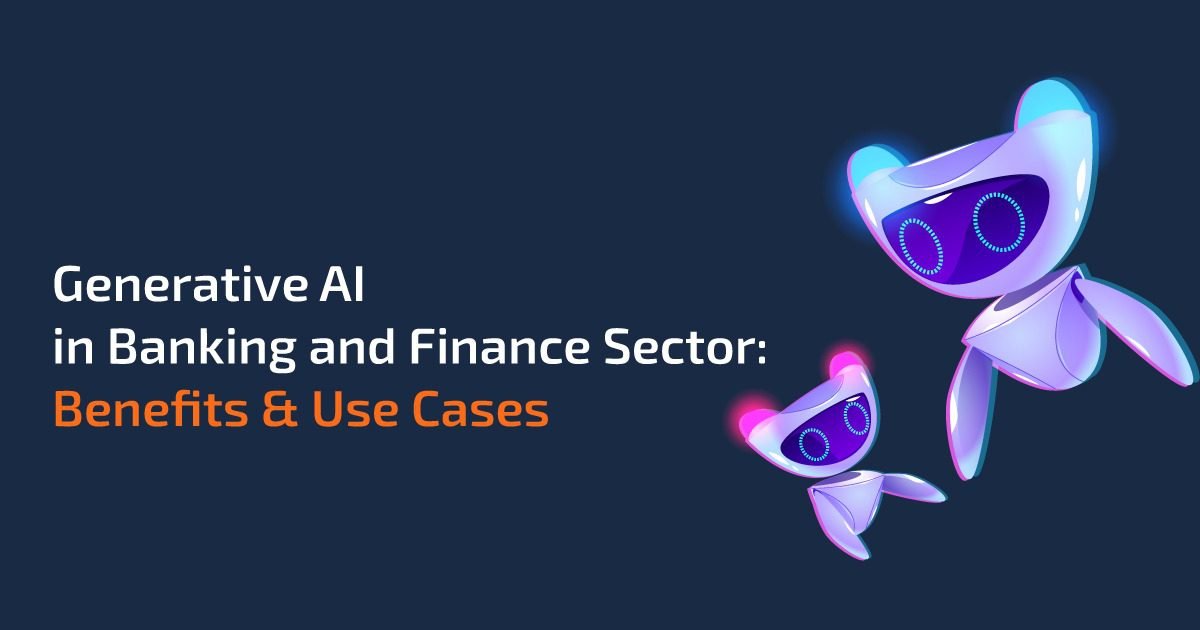
The banking sector has experienced several technological disruptions, and the most recent one to hit the scene is generative AI. Generative AI is a cutting-edge machine learning system that can analyse vast volumes of data and generate original knowledge and content from it. By utilising financial data at scale, it enables bank management to take educated choices, offer individualised services, evaluate risks, and more.
According to McKinsey, generative AI may be able to save the banking industry up to $340 billion yearly, depending on its level of adoption. With less human error and lower operating expenses, technology improves banking operations. More crucially, generative AI development services enable banks to communicate with customers personally without relying too heavily on staff.
How AI is Transforming the Banking Sector
Financial institutions compete in an established industry where just a few things set them apart from one another. The rise of AI widens the field of competition. Banks are well-positioned to use AI to gain a competitive edge, offer appealing products, and expand their client base. A poll found that 77% of bankers concurred that the capacity to fully utilise AI is essential for organisational survival in the banking sector.
Banks are able to put into practice initiatives with a broad impact thanks to the development of cloud infrastructure, storage options, and security technology. The ramifications of AI adoption, especially those driven by powerful language models, will, in my opinion, centre on improving consumer experience. For instance, the Deutsche Bank employs AI to provide pertinent recommendations based on recognised risk indicators to assist clients in managing their investment portfolios.
By making it easier to access diverse financial products and services, generative AI allows conversational banking. Such technologies use machine learning models that have been taught to comprehend and communicate in natural human languages. As a result, banks may broaden the product range they provide to various geographic areas while still providing accurate information and assistance to those consumers.
The automated elements that AI introduces to the traditional banking procedure will be advantageous to banks as well. With AI, banks can be accessible to customers around-the-clock on a variety of channels to respond to their questions and address problems. In this approach, AI aids human support staff in responding to typical inquiries, freeing up the latter to concentrate on complicated issues.
Common Applications for Generative AI in Banking
In the banking sector, there are many opportunities for interpersonal contact. And this offers generative AI tremendous opportunity to make significant improvements. I include a few real-world usage scenarios below.
Chatbots
The majority of banks now use chatbots to handle consumer enquiries. Bankers may use sophisticated language models that can communicate like humans thanks to generative AI. Customers may obtain quick answers by asking questions like “how much is my balance” or “change my billing address” rather than having to sort through a menu of options.
Portfolio Management
Banks may use generative AI to take a more precise approach when advising consumers on portfolio strategies. Large-scale economic data is used to train the deep learning model initially. Then, to create a suitable portfolio, bankers employ the AI system to forecast future trends based on a variety of shifting financial variables, such as currency rates, inflation, and political dynamics. Customers are not need to publicly reveal their financial situations in order for this to occur, which creates a more cosy and private banking atmosphere.
Compliance Management
Banks are under continual pressure to adhere to strict laws in a sector that is heavily regulated. This entails keeping an eye on transactional activity, compiling pertinent data, and promptly presenting it to the appropriate departments. The burden of compliance management will be reduced by using an AI system trained on such procedures. For instance, before approving an account, bankers utilise generative AI to examine client data and make sure they are in compliance with the Know Your client (KYC) Act.
Financial Analysis And Forecasting
Banks, like other companies, must plan ahead to be competitive in changing market conditions. They can perform simulations, forecast economic trends, and modify their positions as necessary thanks to generative AI. For instance, banks may use AI to anticipate the pace of inflation over the next several years and alter interest rates accordingly.
Financial Advisor
Banks can engage each consumer equally and uniquely thanks to generative AI. Before recommending goods that could be of interest to the consumer, the deep learning model evaluates their past data, spending patterns, and risk tolerance. This raises sign-up rates and aids in keeping current clients.
AI-Based Fraud Detection
Banks are under pressure from regulators to protect consumers’ interests and stop fraud attempts since data breaches are so common. Using massive amounts of financial transactions, generative AI may be trained to spot unusual patterns and issue fast alarms. This enables banks to prevent questionable transactions and preserve client confidence.
Loan Score Management
Before granting or denying a loan application, bankers take into account a number of factors. By examining the applicant’s financial history and recent data, generative AI helps credit rating. For instance, you may use the applicant’s salary, age, employment, house, and other credit indicators to train the machine learning model to forecast the chance of a default.
Automation Of Back-Office Processes
To run back-office operations like document scanning, employee identification verification, and safeguarding networking infrastructure, banks make significant investments in their employees. Generative AI integration eases part of the workload for the operational crew. For instance, they may scan, analyse, and categorise physical documents in safe cloud storage using NLP software.
Financial Report Generation
Machine learning models that can display organised data are the foundation of generative AI. This enables banks AI systems to instantly produce financial statements using automation. Customers may, for instance, ask for personalised cash flow or income reports, which the AI turns into files in a matter of seconds.
Legacy Software Maintenance
Some banks continue to use software created with out-of-date programming languages. Developers employ generative AI and the underlying big language models to produce the code rather than completely redesigning the software. When moving the software to a more recent programming framework, this increases coding effectiveness and lowers human error rates.
AI’s Advantages in the Banking and Finance Sector
After incorporating AI into their customer-facing and internal business operations, banks have already experienced significant advantages. Some of them are:
- Improved judgment. With the use of AI-enabled insights, bankers, fund managers, and other financial players support their choice. In erratic market situations, they employ AI algorithms to minimise risks and maximise possibilities.
- Personalised client interactions. Customers are no longer subjected to generic product pitches or lengthy support wait times. Instead, consumers receive rapid service and always have access to relevant information through websites, apps, and other digital channels.
- Increased effectiveness. Financial organisations can increase operations and get over bottlenecks that hinder human procedures thanks to AI technology. They automate time-consuming and repetitive operations using AI technology, freeing up the banking personnel to provide greater value to consumers.
- Stronger security. AI is used by financial organisations to protect clients from data dangers. AI also enables banks to respond quickly to online threats by identifying and halting fraudulent activity in real time.
- Enhanced risk control. AI analyses the large amount of financial data accessible to banks to help them better manage risks. Instead of jumping to conclusions, bankers use predictive insights to safeguard assets, overcome obstacles, and seize market opportunities.
- Regulatory compliance supported by AI. AI is used by banks to continually evaluate their dedication to complying with regulatory obligations. Tasks that bank officials used to perform are now automated by AI systems, which saves institutions money and keeps them out of serious trouble.
- Enhanced privacy. When customers discuss their financial alternatives with AI, they feel more secure and at ease. They have the option to withhold personal information and ask that all conversational data be deleted after the session if they so want.
What Limits Generative AI in Finance and Banking
There is little doubt that banks and lending institutions may gain from generative AI. But for financial use cases, the sector must address specific issues and proceed cautiously with generative AI.
Data Quality
For banks to scale generative AI systems, access to vast quantities of high-quality training data is necessary. Before they are prepared to carry out activities particular to the generative AI banking industry, foundational models, or deep learning models, must be taught. The final AI system won’t operate as planned if the training data is not accurate, full, relevant, or huge enough.
Privacy And Security
A significant quantity of data must be stored and sent via the network infrastructure while training generative AI models. Banks may be prohibited by financial restrictions from utilising certain data for deep learning model training. Additionally, while developing or using generative AI systems, banks must manage data concerns in order to safeguard the privacy of their clients.
Numerical Accuracy
(At least initially) Arithmetic computations are not intended for generative AI. When executing mathematical operations on existing operations, calculators follow a set of rules. Meanwhile, generative AI models provide fresh, perhaps inaccurate responses. Therefore, it seems sense to implement safeguards, such human approval, to stop inaccurate findings from impairing banking operations.
Future Of Generative AI in Banking
- The use of generative AI will change how banks function and interact with their clients. With ChatGPT, we can already observe how generative AI is affecting more general customers.
- By typing particular prompts, users of ChatGPT may narrate tales, write programme code, make lyrics, and more. The banking sector will also observe comparable use cases modified for financial processes
- When generative AI is adopted across the institution, both bankers and consumers will profit from its efficiency and personalisation. However, banks must first have a sound strategy for implementing AI.
How Can Banks Transform to Become AI-First?
Banks that want to fully utilise generative AI must make sure they have the organisational capacity to grow the machine learning technology. Banks must combine diverse divisions and train people in AI-friendly skills, beliefs, and mindsets rather than continuing to run their businesses in silos. For instance, banks retrain employees or recruit AI specialists to keep current with new AI technology.
In order to enable AI systems, banks must next review their technical infrastructure and capabilities. Large volumes of data need to be stored securely in order for generative AI models to be trained and used. Additionally, banks must decide whether their current technological capabilities are enough and whether it is possible to combine internal AI skills with outside services.
Banks use generative AI models to help decision-making across several domains once the technology is in place. Such steps ought to be practical, with accurate risk assessment and initiatives to keep the AI models getting better. For instance, they use AI to supplement repetitive or labor-intensive operations like loan approval or customer support.
Banks must reconsider their approaches to client interaction if they want AI to have a significant influence on customers. Banks should reconsider how AI might be incorporated to offer additional value for consumers rather than concentrating on certain goods. For instance, Tally employs AI algorithms to assist clients with managing credit card payments and quickly reducing debt.
Tips On Using Generative AI in Banking
Despite the excitement, there are still several obstacles in the way of deploying generative AI in banking use cases. I offer a number of strategies for making such adjustments more tolerable.
- Determine which particular banking procedure gains the most from generative AI. Before expanding the AI system to different use cases, it must be trained, deployed, and tested. Before pushing the technology to important use cases like credit scoring, for instance, you train an AI chatbot to serve the customer service team.
- Make that the proper data protection procedures are in place to preserve consumer privacy and adhere to industry standards. Encrypting the data that generative AI utilises, for instance, avoids abuse in the case that they are unintentionally exposed.
- The calibre of the training datasets has a significant impact on how well the generative AI model performs. Therefore, make sure the data are large enough to represent the target demographics and are labelled correctly.
- Use generative AI with caution because it is not yet ready for exact arithmetic computations. Install the necessary protections to stop inaccurate results from negatively harming consumers.
- A generative AI model calculates based on input. Provide the model with particular consumer financial information, such as prior transactions, financial objectives, and risk tolerance, when utilising it to customise the banking experience in order to deliver pertinent results.
Summary
With personalised and effective services, banks will engage clients differently as a result of generative AI. When properly implemented, it has the potential to save financial institutions a significant amount of money. Generative AI plays a huge role in the finance sector, whether it is via identifying fraudulent transactions or enhancing customer care with chatbots.